Real-world data for diabetes treatment and prevention
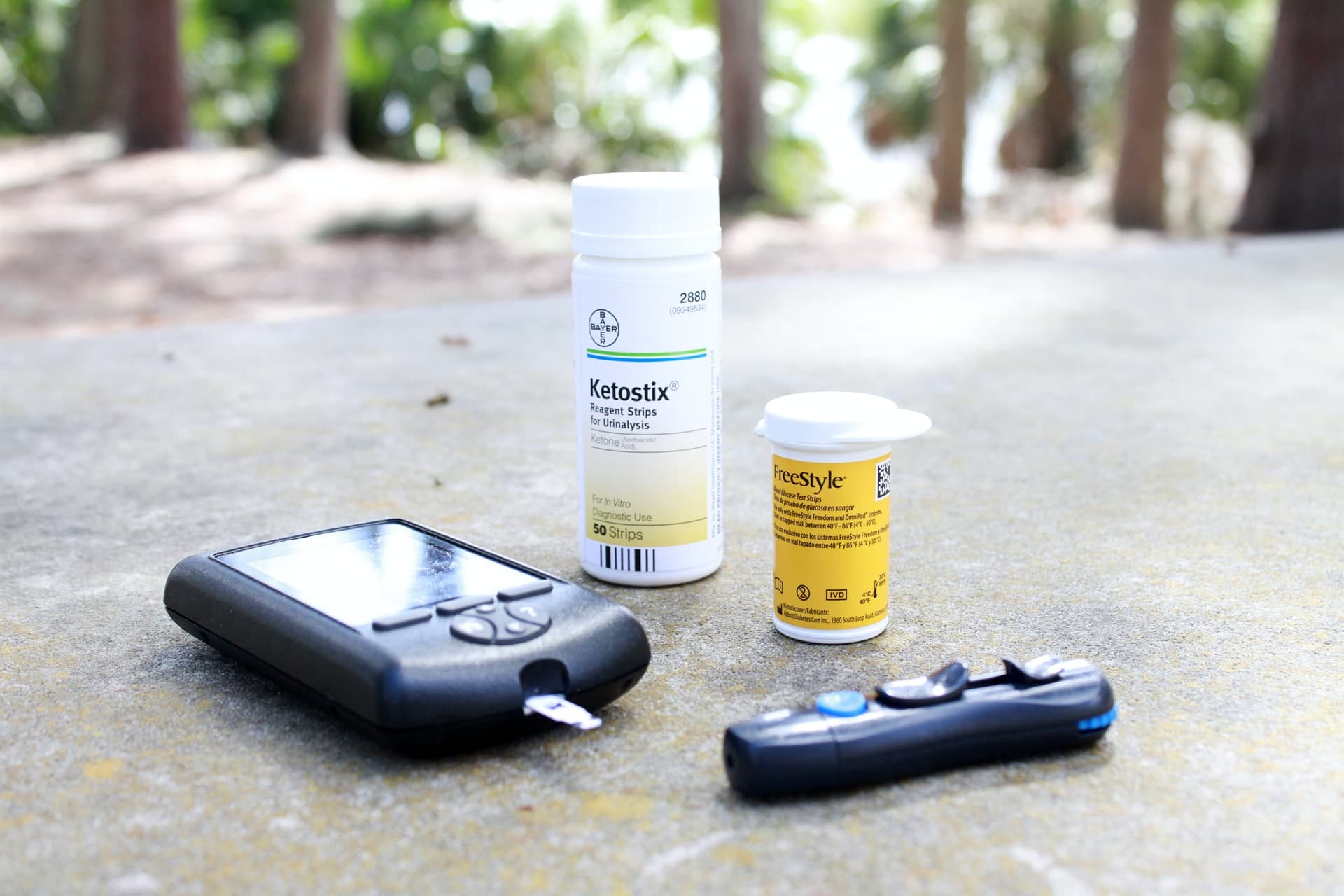
Type 2 diabetes is arguably among the most prevalent diseases in the world. According to the World Health Organisation the number of diabetes patients “has risen from 108 million in 1980 to 422 million in 2014.” [1] The cost of the continuously growing diabetes epidemic is skyrocketing to more than $850 billion US-Dollar a year. [2] Technology-driven solutions have the potential to lead an effective and preventative approach to combat diabetes. The key to early detection of diabetes: real-world data from wearable devices and medical sensors.
Trackable indicators in diabetes
Wearable devices can help to track real-world data relevant to identify diabetes patients in a reliable and actionable way. Common fitness trackers and smartwatches are equipped with a wide array of sensors enabling the holistic assessment of a patients’ health. Besides the obvious data points, namely blood glucose, the main indicators for increased diabetes risk are resting heart rate (RHR), physical activity, and sleep patterns.
In a study analyzing consumer-grade wearables, researchers found that “the correlation between wearable metrics and fasting glucose suggests that wearables may provide an early indication of increased diabetes risk“. Wearables, in particular, proved to be “equivalent to—if not better than—clinical RHR measurements.”[3]
Physical activity – technology providers like Thryve aggregate wearables sensor data into such indices – proves to increase the risk of adverse health conditions, including non-communicable diseases like diabetes. Researchers analyzing data from Fitbit devices noted that “individuals with chronic diseases exhibit reduced activity levels compared with healthy populations.“[4]
When adding sleep data measured by wearables to the analysis researchers found that „decreased physical activity and sleep leads to worsening glycemic control among patients with diabetes“.[4]
Real-world data provided by Thryve
Thryve’s technology enables access to real-world data from hundreds of wearables, medical devices, and consumer health data silos. We unify patients’ data from smartwatches, blood glucose meters, and many other sources into more than 300 harmonized, digital data points. We integrate directly into existing apps or enable to access data via our clinical research infrastructure.
Thryve helps you to get data on activity, sleep, cardiovascular information, nutrition, temperature, respiration, body composition, blood tests, environmental (e.g. weather, pollution), wellbeing, and intestinal flora.
Developing digital biomarkers for type 2 diabetes mellitus screening
Digital biomarkers have the potential to transform diabetes prevention programs. First clinical studies have proven that wearables have to potential to enable the prediction of developing diabetes mellitus type 2 (T2D).
Today’s prevention efforts lack objective data inputs of patients. Self-reported patient behavior proves to be inaccurate. Wearables can help: “Measuring correlations of wearable data and patient-reported outcomes increase transparency of patient monitoring. […] utilization of data-driven, mechanical devices, such as wearables, can decrease self-reporting bias, enhance data integrity, and reduce reproducibility issues between studies.“ [4]
As wearables provide continuous, high-density data on different vital and activity parameters they prove to be of incredible value: “[…] findings suggest that it may be possible to use continuous or periodic self-monitoring of individuals at risk of T2D, specifically those in a prediabetes state, for screening and early detection of disease progression. This is particularly important as evidence shows that reversal of T2D is possible, with a higher success rate when interventions are undertaken within the first 5 years of the disease.” [5]
Leveraging photoplethysmography (ppt) can be a different approach to develop digital biomarkers for diabetes assessments. Thryve’s technology enables easy access to ppt data for researchers. The use of basic smartphone cameras has proven to be a “readily attainable, non-invasive digital biomarker of prevalent diabetes.” [6] These findings were confirmed by researchers stating: “The analysis of PPG signals can provide a simple, inexpensive, and noninvasive means for studying diabetes development. By that means, PPG can assist hbA1C; or it can be used as an alternative measure as an early detection of diabetes progression”. [7]
[1] WHO. Fact-sheet Diabetes. HTTPS://WWW.WHO.INT/NEWS-ROOM/FACT-SHEETS/DETAIL/DIABETES
[2] Reuters. Cost of diabetes epidemic reaches $850 billion a year. HTTPS://WWW.REUTERS.COM/ARTICLE/US-HEALTH-DIABETES/COST-OF-DIABETES-EPIDEMIC-REACHES-850-BILLION-A-YEAR-IDUSKBN1DD2SW
[3] Lim WK, Davila S, Teo JX, Yang C, Pua CJ, Blöcker C, et al. (2018) Beyond fitness tracking: The use of consumer-grade wearable data from normal volunteers in cardiovascular and lipidomics research. PLoS Biol 16(2): e2004285. HTTPS://DOI.ORG/10.1371/JOURNAL.PBIO.2004285
[4] Weatherall, J., Paprocki, Y., Meyer, T. M., Kudel, I., & Witt, E. A. (2018). Sleep Tracking and Exercise in Patients With Type 2 Diabetes Mellitus (Step-D): Pilot Study to Determine Correlations Between Fitbit Data and Patient-Reported Outcomes. JMIR mHealth and uHealth, 6(6), e131. HTTPS://DOI.ORG/10.2196/MHEALTH.8122
[5] Lam B, Catt M, Cassidy S, Bacardit J,Darke P, Butterfield S, Alshabrawy O,Trenell M, Missier P
Using Wearable Activity Trackers to Predict Type 2 Diabetes: Machine Learning–Based Cross-sectional Study of the UK Biobank Accelerometer Cohort. JMIR Diabetes 2021;6(1):e23364. HTTPS://DOI.ORG/10.2196/23364
[6] Avram, R., Olgin, J.E., Kuhar, P. et al. A digital biomarker of diabetes from smartphone-based vascular signals. Nat Med 26, 1576–1582 (2020). HTTPS://DOI.ORG/10.1038/S41591-020-1010-5
[7] Qawqzeh, Y. K., Bajahzar, A. S., Jemmali, M., Otoom, M. M., & Thaljaoui, A. (2020). Classification of Diabetes Using Photoplethysmogram (PPG) Waveform Analysis: Logistic Regression Modeling. BioMed research international, 2020, 3764653. HTTPS://DOI.ORG/10.1155/2020/3764653