How Sleep-Measuring Wearables Transform Rest and Recharge
Sleep isn’t just another biomarker; it’s a powerful keyhole into our physical and mental well-being, the very cornerstone of our health. 🛌
Its influence on physical health, mental clarity, and emotional balance is undeniable. With the advent of wearable technology, tracking sleep patterns has become more accessible and convenient than ever before. Wearables, ranging from smartwatches to dedicated sleep trackers, offer insights into our nightly rest, shedding light on sleep duration, quality, and even potential disturbances. However, it’s important to acknowledge that while wearables offer valuable insights, relying solely on them can result in inaccuracies that might distort our understanding of our own sleep patterns.

Sleep Debt
Sleep debt, often known as a sleep deficit, emerges when the amount of sleep needed doesn’t match the amount received. This can lead to serious health consequences. For instance, research indicates that individuals consistently getting less than 7 hours of sleep per night have a 20 – 32% higher risk of developing cardiovascular diseases. Additionally, insufficient sleep weakens immunity, disrupts metabolism, increases the likelihood of accidents, and impacts memory and cognitive function.
Sleep Studies
Studying sleep using wearables for research and studies offers the advantage of capturing real-life sleep patterns and behaviours over an extended period. Wearables provide non-intrusive monitoring in participants’ natural environments, allowing researchers to collect objective data on sleep quality and trends. These devices enable large-scale data collection, minimise disruptions to sleep routines, and offer insights into the impact of daily life on sleep. This approach enhances the understanding of sleep’s role in overall health and well-being.
In a recent study using Whoop as a data source, which we’re now rolling out as an integration, sleep data was compared with established sleep monitoring methods like polysomnography, the ‘gold standard’ for monitoring sleep. The findings, which included a remarkable 95% confidence interval in sleep data when compared to polysomnography, showed that wearables can indeed improve sleep quality and accurately measure sleep and cardiorespiratory factors. This statistic underscores the reliability and effectiveness of wearable technology in sleep research, further highlighting its potential for advancing our understanding of sleep-related health.
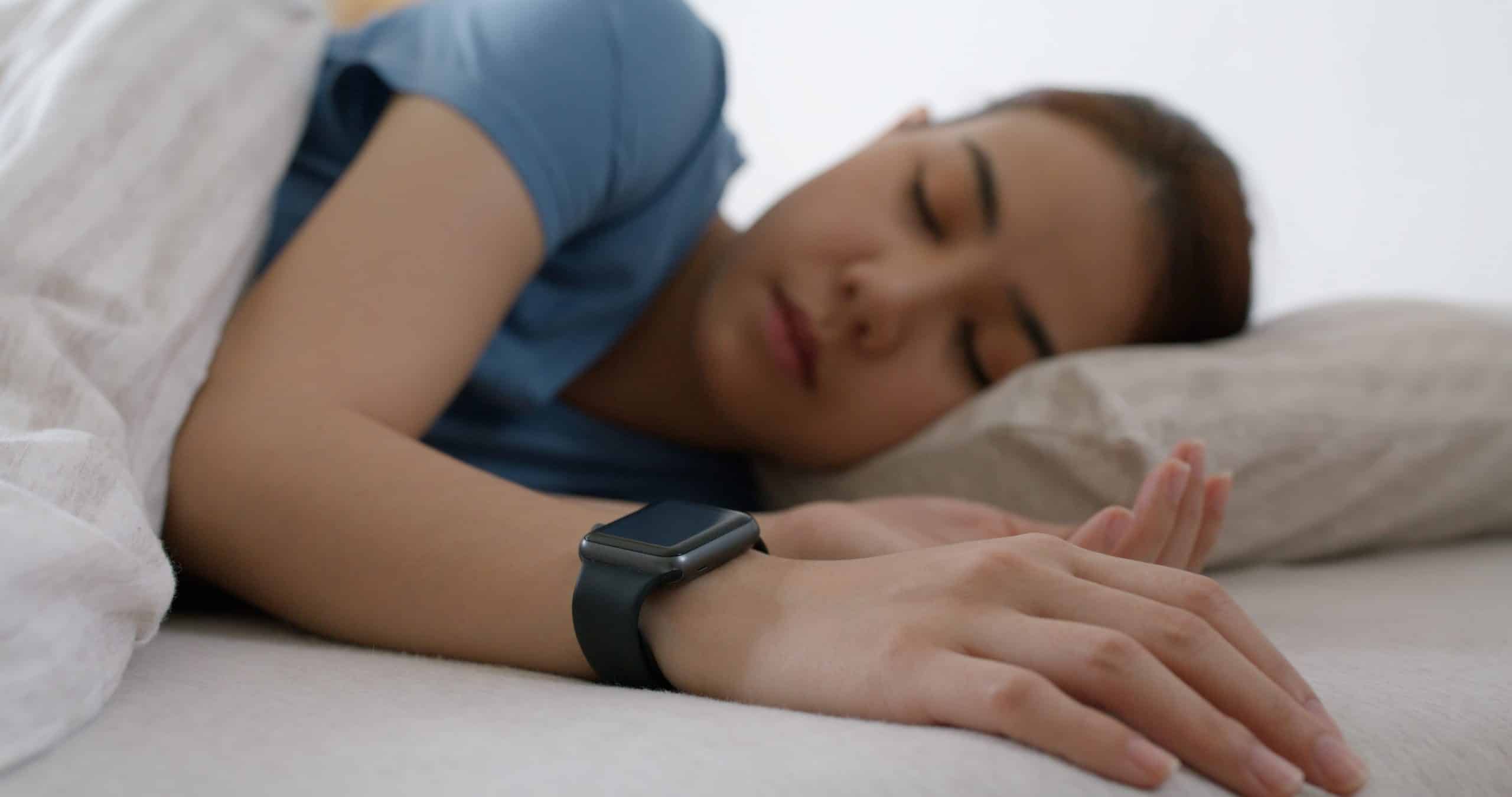
Navigating the Maze of Sleep Tracking Metrics
Tracking sleep accurately poses challenges due to the diverse nature of devices used for this purpose. Each device relies on varying sensors and algorithms, making it challenging to establish a universal standard for measuring sleep. Moreover, several factors like device placement, individual sleep habits, and the complexity of sleep cycles further complicate consistent and precise tracking across different devices. Other key factors include:
➡️ What’s your actual sleep time per calendar day?
Some devices simply sum up sleep time per calendar day. As most individuals have split sleep patterns (e.g., morning and evening sleep), the resulting sleep metric is not fit for use. Resolving this issue of oversimplified representation requires a more nuanced approach, like considering a division of sleep time at noon of a given calendar day.
➡️ How do you account for naps in daily sleep?
Naps, a common component of many people’s sleep routines, are either not adequately accounted for or inaccurately tracked by certain devices, leading to incomplete sleep analysis.
➡️ How to resolve deviating data from multiple sources?
The use of multiple sources for tracking sleep frequently introduces vast complexities in data handling. Using several devices to record sleep (e.g. coupling your Apple Health account and Watch with an Oura Ring) typically leads to deviating sleep states and times per source, making it tremenduously challenging to create a unified sleep record and interpretation across devices.
➡️ How to differ between bedtime and sleep?
A significant challenge arises from discrepancies between going to bed and actually falling asleep. Devices that rely on detecting bedtime or relaxation times might not accurately reflect the moment an individual truly falls asleep, potentially distorting the overall accuracy of sleep data.
➡️ How to track sleep from users across timezones?
We live in a global world and people eat, sleep and travel across countries and timezones. Many sleep-tracking devices provide data with a reference to the timezone or timezone difference to a given standard (like UTC – Coordinated Universal Time). To get reliable sleep metrics per user and day, it’s essential to reliably consider timezones and avoid skewing daily sleep data for individuals who travel or work across time zones.
Thryve to the Rescue
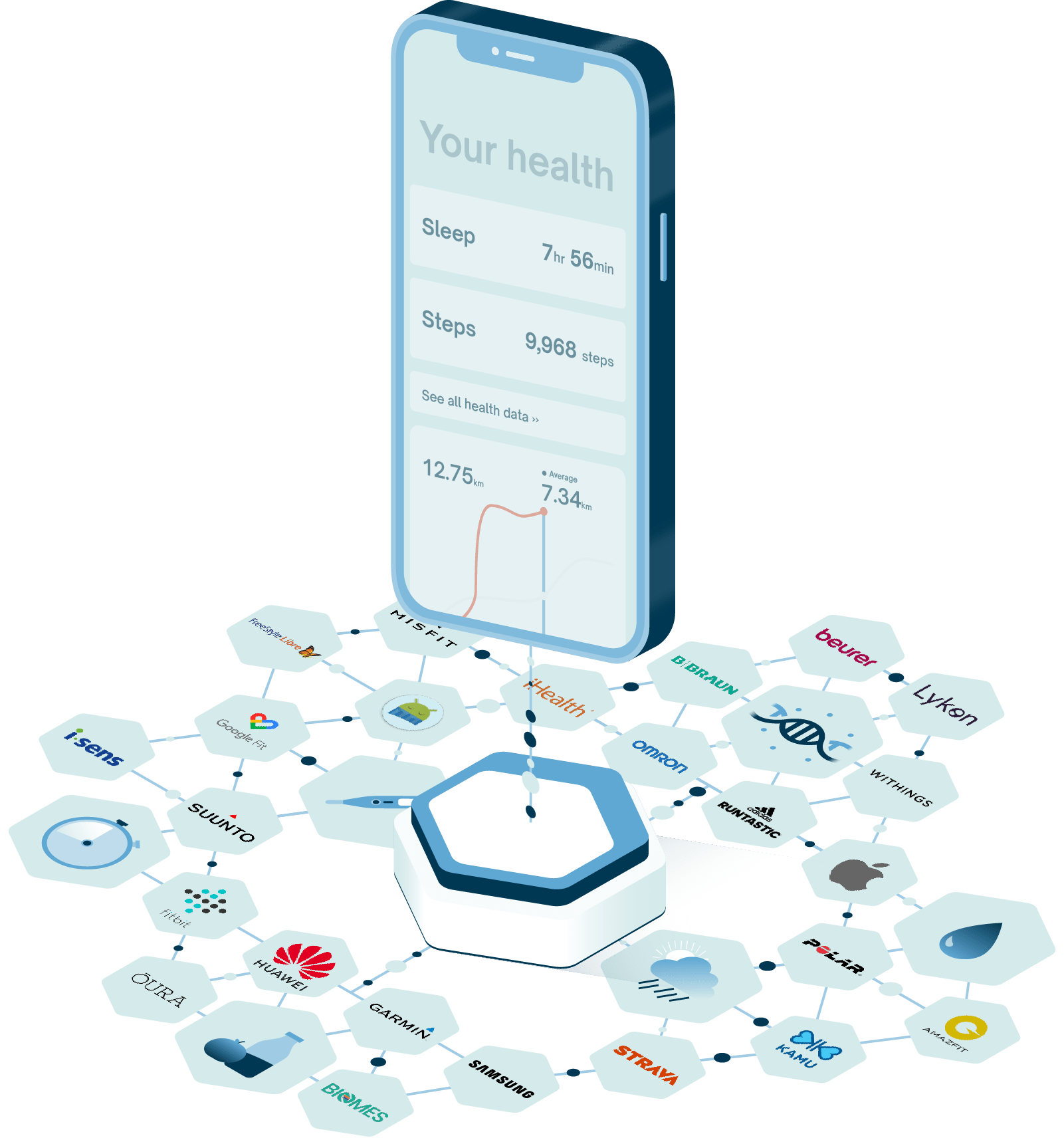
Sleep measurement differs significantly from other trackable data points, primarily due to the unique implementations of sleep tracking across various devices and data sources. One of the main sources of confusion stems from the fact that each tracker or data source tends to use its own set of terms for the same concepts. This leads to variations in the number of sleep stages (ranging from 3 to 5) and differing names for identical elements (like “core sleep” in Apple devices and “light sleep” in Garmin devices, for example)
While steps or heart rate readings can be assessed as either accurate or inaccurate, the assessment of sleep is more intricate and nuanced. Recognising this challenge, we strive to gather all available sleep data and harmonise it as closely as possible for meaningful comparisons.
So how do we tackle these issues?
✅ We roll up our sleeves and dive into the nitty-gritty of source APIs and documentation, mapping each data source semantic onto the unified Thryve data structure.
✅ We cover time zones for sleep timing, providing a reliable metric towards sleep start and end times.
✅ We unify sleep metrics across devices, providing a reliably unified sleep data set per day.
✅ We crunch the numbers to generate statistics like sleep efficiency (total sleep / (total sleep + awake time)) and how long it takes to fall asleep (latency).
And we don’t stop there – the Thryve engine serves complex metrics like Sleep Regularity, which we generate based on several days of sleep epoch data.
Trusting Thryve with your sleep data analysis means gaining clear insights and precision, allowing you to confidently understand your sleep patterns and make informed choices for your well-being.